An equilibrium system is rather often only an abstract idealization.
Typically, a given system is not in equilibrium with its environment, but is exchanging matter and/or energy with it; fluxes are present in the system. The usual
way to describe the physics of such a system is to write down a master equation for the time-dependent probability that a given mesoscopic state is realized at time
t. The physics is then embedded in the transition rates between these mesoscopic
states. In the long-time limit the system eventually settles into a steady-state and,
depending on the values of some control parameters or the initial configuration,
different nonequilibrium steady states can be reached, and sometimes a
breaking of ergodicity takes place. Upon varying some control parameters the system may change its steady state and this is called a nonequilibrium phase transition (Hinrichsen, 2000).
References:
One of the original, and still primary reasons for studying networks is to understand the mechanisms by which diseases, information or rumors spread over. The heterogeneity of the connectivity distribution in scale-free networks greatly impacts the dynamics of processes that they support.
One of the most remarkable examples is that in uncorrelated SF networks with a connectivity exponent γ ≤ 3, epidemic processes do not posses an epidemic threshold below which diseases cannot produce a macroscopic epidemic outbreak or the development of an endemic state. This feature, observed in several epidemic models (Pastor-Satorras & Vespignani, 2001; Moreno, 2002; Newman 2002), is deeply rooted in the presence of very large connectivity fluctuations in infinite SF networks. The change in the behavior of the processes is so radical in this case that it has been claimed that the standard epidemiological framework should be carefully revisited. This might be bad news for epidemiologists, and those fighting natural and computer viruses.
On the other hand, in a number of important technological and commercial applications, it is desirable to spread the “epidemic” as fast and as efficient as possible, not to prevent it from spreading. Important examples of such applications are epidemic (or rumor-based) protocols for data dissemination and resource discovery on the Internet (Vogels, 2002), and marketing campaigns using rumor- like strategies (viral marketing).
The
susceptible-infected-susceptible (SIS) [8, 9], susceptible-infected-removed (SIR) [10, 11], and susceptible-infected
(SI) [12, 13, 14] models on complex networks have been
extensively studied recently.
Epidemic model SIR
- Individuals are either susceptible (S), Infected (I) or removed (R).
- Susceptibles become infected through their contacts with infected individuals at
a rate β.
- Infected individuals are removed (die out) at a rate γ.
- There is an epidemic threshold above which the diseases spread through the population:
β/γ > λC
However, this result is based on the homogeneous mixing hypothesis (complete graphs):
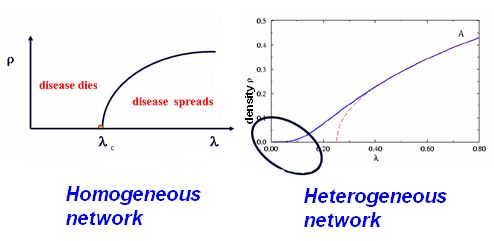
Selected papers:
- Epidemic Spreading in Scale-Free Networks
R. Pastor-Satorras, and A. Vespignani
Phys. Rev. Lett. 86, 3200 (2001).
- Epidemic outbreaks in complex heterogeneous networks
Y. Moreno, R. Pastor-Satorras, and A. Vespignani
Eur.
Phys. J. B 26, 521 (2002).
- Spread of epidemic disease on networks
M.E.J. Newman
Phys. Rev. E, 66, 016128 (2002).
- Dynamics of Rumor Spreading in Complex Networks
Y. Moreno, M. Nekovee, A.F. Pacheco
Phys. Rev. E, 69, 066130 (2004).
- The Power of Epidemics: Robust Communication for Large-Scale
Distributed Systems
W. Vogels, R. van Renesse and K. Birman
in the Proceedings of HotNets-I,
Princeton, NJ (2002).
|